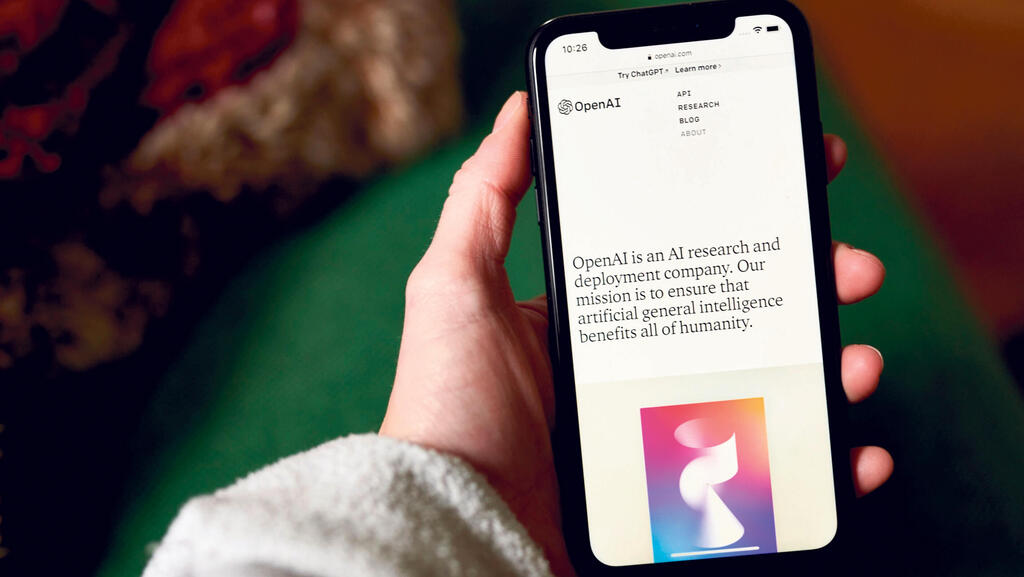
Beyond bigger models: How AI’s next era will rethink growth
Scaling up is no longer the answer; AI companies pivot to refining existing technologies.
When OpenAI launched ChatGPT to the general public two years ago, it amazed the world with the chatbot's conversational capabilities and raised fears about a future where artificial intelligence (AI) could threaten humanity. Concerns intensified in March 2023 with the launch of GPT-4, a Large Language Model (LLM) that significantly enhanced ChatGPT’s abilities. "Mitigating the risk of extinction from AI should be a global priority," warned senior AI figures, including OpenAI CEO Sam Altman, in May of that year.
Yet, something surprising happened: the anticipated AI breakthrough didn’t come. While other companies like Google and Anthropic launched models comparable to GPT-4, and OpenAI rolled out upgrades such as GPT-4o, the next leap in AI capabilities failed to materialize. The methods that drove generative AI’s (GenAI) recent breakthroughs have hit a ceiling, unable to push the field to the next level.
A "much smaller" improvement
OpenAI’s development of Orion, the anticipated successor to GPT-4, has reportedly encountered obstacles. Researchers within the company have described the improvement in performance as "much smaller" than the leap from GPT-3 to GPT-4. "Orion is not reliably better than GPT-4 at handling certain tasks," researchers told TechRadar, with coding being a particular challenge. However, they noted that the model's language skills are stronger.
One critical issue is the shortage of high-quality training data. GPT-4 and similar models were trained on vast amounts of freely available online content, but subsequent models lack access to significantly new sources of data. This limitation constrains their ability to improve meaningfully.
Training these increasingly complex models also involves enormous costs, requiring tens of millions of dollars for AI chips and energy. Hardware systems are growing more intricate and prone to failures, with some companies even struggling to power their training arrays.
OpenAI co-founder Ilya Sutskever, who left the company earlier this year to establish Safe Superintelligence, has acknowledged the limits of current LLM training methods. "The concept of bigger is better has reached its limits," he told Reuters. "The focus now is on scaling up the right things. Everyone is looking for the next breakthrough."
Faced with these challenges, companies are shifting focus to improving existing models. Techniques such as test-time compute enable enhancements during use by generating multiple responses and selecting the best one. OpenAI applied this approach to its o1 model, unveiled in September, which excels in tasks like programming and scientific reasoning by taking more "thinking" time before responding. Competitors such as Anthropic, Google’s DeepMind, and Elon Musk’s xAI are also developing similar techniques.
Related articles:
This pivot could have broader implications for the AI ecosystem, particularly the AI chip market. Over the past two years, Nvidia's dominance has been fueled by demand for its chips to train massive AI models. However, the realization that bigger models no longer yield exponential improvements could reduce demand for training chips. Instead, the focus may shift to chips optimized for running models, an area where companies like Intel could gain ground.
As AI companies pivot from scaling to refining, the field enters a new phase of innovation. While the breakthrough moment may be delayed, the drive for more efficient and effective methods could reshape not just AI but the industries supporting its rapid evolution.