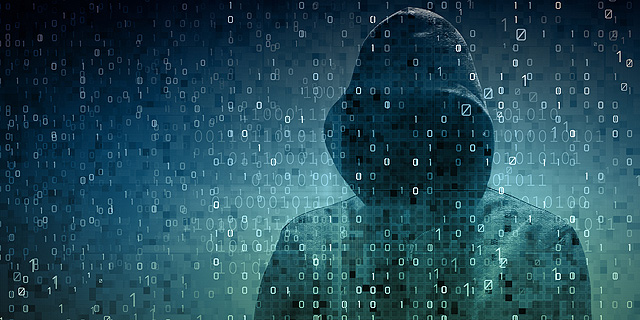
Opinion
Current Privacy Legislation Fails in Defining Sensitive Data
Researcher Dov Greenbaum suggests a new model categorizing personal data according to how accurately it describes an individual, even without disclosing their identity
Not a day goes by without yet another cybersecurity breach and thousands, if not millions, of personal data records are leaked. In some instances, the hackers are just small, sometimes foolish, felons, that are easy to catch. One hacker who recently made off with data belonging to 100 million Capital One Credit Card customers posted about some of her ill-gotten gains on her GitHub page, which included her full name.
However, since we cannot always rely on simpleton scofflaws, the law itself needs to be robust. Much of the law relating to the safeguarding and protection of data and privacy focuses on an archaic distinction between sensitive data (and its myriad synonyms) and non or less-sensitive data. According to standard operating practices in many jurisdictions around the world, sensitive data must be well protected. The less-sensitive, not so much.
But this distinction is problematic. Recent research in the areas of predictive analytics and data mining strategies continues to show how sufficient personal data of the less sensitive variety can be used to reveal sensitive personal information, including genetic and medical information.
In an early example, a group of researchers from the University of Texas was able to extract personal information from an anonymized Netflix dataset that was distributed in an effort to enhance the Netflix recommendation algorithm.
In a more recent example, last month, 99.98% of an anonymous dataset was de-anonymized by a U.K. academic group using non-sensitive and easily available personal data like age, gender, and marital status.
This increasing ability to deanonymize information is especially problematic, as even the relatively legally onerous pan-European General Data Protection Regulation (GDPR) allows for less stringent data protections for anonymized sensitive personal data. The recent scholarship, which shows just how easy it is increasingly becoming to de-anonymize even extensively anonymized datasets by machine learning, suggests that the GDPR’s flexibility here might be misguided.
The standard legal divisions described above, which are broadly implemented in many jurisdictions with the goal of safeguarding particularly personal information, and are now cemented most pervasively by the GDPR, are not only increasingly nonsensical and ineffective, they are also costly and inefficient in terms of compliance and judicial oversight.
Even the recent California Consumer Privacy Act (CCPA), while it has a more progressive definition where protected personal information is defined as information that “could reasonably be linked, directly or indirectly, with a particular consumer or household,” remains problematic. Technological progress has made its vague terminology a moving target which is good for neither the consumer nor the database owner.
As a result of the emerging reality, in which there is no real distinction between various bits of data, new data protection paradigms need to be developed that will adequately prevent the malicious misuse of personal information.
To this end, I propose a tripartite distinction that seeks to minimize compliance costs for database owners, while also adequately protecting the interests of consumers, patients, and other individuals’ data. It is also vital to refocus away from the primacy of identification in favor of a paradigmatic distinction between identifying data and descriptive data.
The best example of such a distinction might be in your genes. The CODIS database employed by the FBI uses stretches of your DNA to identify you and to distinguish you from every other human on the planet. Importantly however, these stretches of DNA are in an area of your genome that does not code for anything. Formerly known as Junk DNA, and now reclassified as non-coding DNA, there is nothing in this DNA that in any way describes you. It is like your government-issued identification number—it identifies you uniquely, but says nothing about who you are. In contrast, a collection of DNA from coding regions (i.e., genes) could say a lot about your physical and mental health or whether you think cilantro tastes like soap.
Clearly, the privacy implications resulting from your identification are significantly less scary than the consequences of your description. As such, a better legal distinction would focus on identity versus description rather than sensitive versus non-sensitive.
The first level of protected data and the most important vis-à-vis privacy considerations would be what standard regulatory bodies typically refer to as sensitive personal data. This type of data should be referred to instead as personally descriptive and would include data on political opinion, physical and mental health, biometric data, criminal background, and religions and philosophical beliefs. This type of data can not only uniquely identify you, but is also very descriptive of an individual, covering both mental and physical traits and providing a window into who that person really is. According to the model I am suggesting, this type of data would require the most onerous regulatory compliance.
The lowest level of protected data would be raw compilations of personal information that relates only to a person’s identity but is not necessarily descriptive. This information would include names, locations, and identity numbers. This type of data, when collected in-bulk and uncorrelated with other more private types of information, would require the least onerous regulatory compliance given the low level of its intrusiveness.
Related stories
The middle level of data would be personal identity data, which comprises curated information that can easily be correlated with other data sets to extract descriptive data. This type of data, which is usually in the lowest category or regulatory oversight necessitates a higher level of protection given that once curated it is relatively straightforward to extract descriptive and thus sensitive personal information from other data sets with which it can be correlated.
How would this model protect you? The data that is employed to re-identify anonymized datasets is often clean and structured, i.e., curated. Raw messy data is a much less useful tool, particularly for the vast majority of not-so-savvy malicious actors. Thus, rather than throwing in the towel and suggesting that all data needs to be protected at an equally burdensome level, this proposal creates a further level of distinction that allows some data to be easily handled, and two higher levels that respectively require increasingly arduous efforts of protection from those that collect and sell our data. That way we don’t all end up on GitHub again.
Dov Greenbaum, JD PhD, is the director of the Zvi Meitar Institute for Legal Implications of Emerging Technologies and Professor at the Harry Radzyner Law School, both at the Interdisciplinary Center (IDC) Herzliya.
No Comments Add Comment