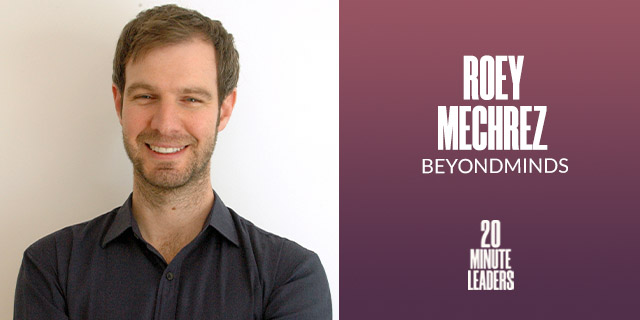
20-Minute Leaders
"The model is important, but it's only one single component"
Roey Mechrez, co-founder of BeyondMinds, talks to Michael Matias about accelerating AI adoption and why it is all about the team
While AI has captured a lot of attention, research, and investment, Roey Mechrez says nearly 90 percent of attempts to put AI into production fail or fail to make a profit. The gap between the potential and the reality prompted Mechrez and his partner to found BeyondMinds. There, they are attempting to bridge the gap between the lab and production by offering companies a platform for AI solutions and transformation. He shares that many companies have a good foundation for working with AI in 2021, but they need faster solutions with a higher success rate for mission critical problems. These solutions are rarely found in off-the-shelf products, as AI needs are usually highly specific. Mechrez believes the team of people around him is a key component to the success of BeyondMinds as they look to help accelerate AI adoption.
Tell me about your background. Why are you so excited about AI in general?
I was an air traffic controller in the Israeli Air Force. Then I did my bachelor’s and master's in biomedical engineering. During my master’s thesis, I was dealing with some computer vision of brain MRIs. Two years after, I found myself doing a PhD in the Technion in general computer vision. I read so many papers and fell in love with the field.
Talk to me about this dissonance from theory to practice.
We founded BeyondMinds with a very significant vision. Rotem Alaluf came from one of the defense companies in Israel with some experience in NLP and putting solutions in production. We identified this gap between the amazing theory and research around AI (and practice). There is a big gap to actually value in practice.
You will find a systematic failure around bringing AI into production or bringing profit out of AI. About one out of ten AI projects or AI POCs actually do production, actually become profitable. There's so much money invested in AI, and about 90% of that money is a waste. It won't lead to ROI. That's what we're trying to solve, bridging this AI theory, lab AI and production.
I'm hearing you hint that part of the reason that these don't get translated into positive ROI is because the whole supply chain is still not easy to maintain or to create yet. Right?
It's a great question. I usually distinguish between the organizational challenges and the technology challenges. I think around 2018, when most companies decided to build a center of excellence and bring AI, they were stuck into a lack of talent, strategy, vendor selection, business alignment, and finding the right starting point. I think at mid-2021, most organizations are already past this maturity level. Now you have a PhD who sits in the lab, you brought them data, and they trained the model, and then what? Can you just take this model and put it in production? The answer is clearly not.
Why is this a difficult problem to solve?
I think the landscape of AI is very interesting. As I see it, you can cut it into three pieces. There are vertical companies, amazing companies that solve a very specific problem. Maybe they use AI as part of their technology, and their product can be delivered off the shelf. Then you have developer tools, companies that develop a tool, a platform; maybe also the cloud providers you can count in this area.
Then, there is BeyondMinds, and we are focusing on delivering business value solutions with AI. Our platform is the enabler to break the failure rate that I described. We are coming with the third element for companies who say, “I want AI in production. I want it fast. And I want a better success rate.” You can come to BeyondMinds and use our platform as an enabler. We're going to customize a silver bullet solution.
AI and data-related problems are characterized by high specificity. I would say 80% of the market cannot be solved by an off-the-shelf product. So companies usually go to their center of excellence and get 18 months delivery time and 10% success. We are providing an alternative path to that awful cycle.
Take me five years down the line. Where does BeyondMinds fit into where the world is moving to?
That's a very complex question. But I think what we're trying to do is to accelerate AI adoption and enable companies to bring more and more AI into various areas of the business. We're not trying to solve one specific use case for the company. We're trying to help them to do AI transformation. The main area that we're working on is dealing with mission critical problems.
AI use cases are shifting from what they call statistical use cases, that's okay that it speaks 80% accuracy. Take for example, Netflix’s recommendation system. It can be better. But it's already bringing value. On the other hand, if you are doing defect detection of iPhone manufacturing, you don't want a bad iPhone to leave the factory.
Do you find that the people that you're working with understand these limitations?
It's improving mainly with business people. Of course, the technical people are facing these barriers daily. I think the people that are actually the customers of these AI solutions, they understand more and more about what AI can offer, what are the challenges, what are the risks, what is required from them.
Business decisions need to take into consideration doing AI development. It's far from, "Here is a data set. Create me a classifier. Let's put that in production." You're going to have different elements that need to be considered. I think that collaboration and mind-set is improving significantly.
What would be the one or two biggest tips you would give to somebody facing these challenges?
Let's start with the easiest one—don't do POC. I think POC is one of the largest traps around AI. Because the gap between the lab and production is so large that the POC is not even relevant. You need to think about production, all the requirements of the production, and how that's going to work and solve that.
Take fraud detection, for example. Five years ago, all the banks had rule-based systems. You might have a rule that a transaction of above $5,000 raises a flag. So, I will do $4,999, right? This problem is so dynamic. Even if you solve it with AI, and you use historical data, next month there will be a new type of fraud. You need to train these models in production consistently. That's a super challenging problem.
A famous element of AI is that when it fails, it fails very silently. You build your dog versus cat classifier, and then you get an image of a giraffe. The model will say that either it's a dog or a cat. It won't tell you that he isn't sure, that he has doubt. It will classify it as a dog. Another element that you need to solve is what you do with out-of-distribution examples. You have the data that you trained your models on, but you will get a weird image that is completely out of the distribution. All of these elements must be solved in production if you're talking about mission critical problems.
I think that's maybe the takeaway that I can give to researchers. The model is important, but it's only one single component. It’s the heart of the system out of a holistic system that needs to be filled to solve production problems.
Next I have three fun questions. Favorite subject in school, K-12?
When I was in seventh grade, I had a carpentry class. Today as a hobby, that's the best thing I love to do. Just bring me a saw and a hammer, and I will be happy.
One of your role models?
My first intuition was to say Michael Jordan because I love the mentality of teamwork that he has. He said something like, "Individuals win games, but teams win the championship." I couldn't agree more. I'm nothing without an amazing team that supports me and builds the company with me, shoulder to shoulder, as a team.
Three words that you would choose to describe yourself?
Teamwork, creativity, and execution.
Michael Matias, Forbes 30 Under 30, is the author of Age is Only an Int: Lessons I Learned as a Young Entrepreneur. He studies Artificial Intelligence at Stanford University, while working as a software engineer at Hippo Insurance and as a Senior Associate at J-Ventures. Matias previously served as an officer in the 8200 unit. 20MinuteLeaders is a tech entrepreneurship interview series featuring one-on-one interviews with fascinating founders, innovators and thought leaders sharing their journeys and experiences.
Contributing editors: Michael Matias, Megan Ryan