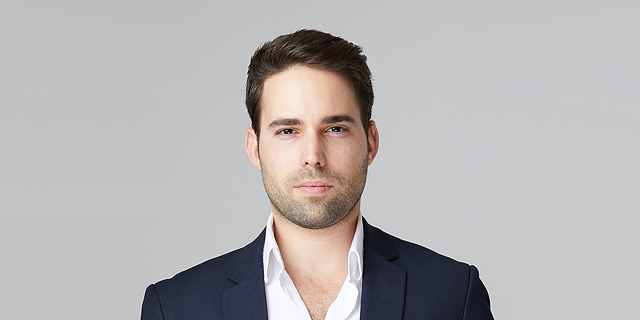
Opinion
If nothing is trustworthy in cyberspace, does AI even have a chance?
“The challenge to embed trust into complex and automated AI-driven processes is a cross-industry phenomenon, as it ultimately stems from AI’s unique and inherent characteristics,” writes Eyal Balicer of Citi
“In God we trust, the rest should be authenticated.”
This W. Edwards Deming paraphrase embodies the ‘zero trust’ sentiment which is gaining increasing traction in the cybersecurity industry.
The prevalence of AI-powered solutions in the cybersecurity domain is only stirring the pot. Afterall, AI models are only as good as the data they rely on (‘garbage-in, garbage-out’), and sensitive AI datasets, which have serious impact on human lives, have been found to be systematically misleading and oftentimes riddled with biases and inaccuracies. This is a slippery slope, as incorrect or corrupted data could even lead to life-threatening incidents in extreme cases.
This phenoenon has been taking roots for a while now and yet governments, companies, and academic scholars, are still struggling to agree on satisfactory tests and guardrails that will ensure trust is inscribed into AI-powered models. Consensus has yet to be reached even around the formation of an acceptable universal metric for measuring AI models’ robustness and soundness, which is a basic requirement for establishing trust and assessing risk in AI-dominant environments.
The challenge to embed trust into complex and automated AI-driven processes is a cross-industry phenomenon, as it ultimately stems from AI’s unique and inherent characteristics, turning model explainability and predictability into a daunting objective for most organizations.
Cybersecurity is different
AI models power a plethora of security products that, inter alia, distinguish between malicious and benign activities, discover vulnerabilities, plot sparse data points onto a single incident timeline, and detect elusive malicious patterns and anomalous behavior. Alongside the demand for elevated precision to counter adversaries’ stealthy and dynamic nature, detection and prevention systems are held to even higher standards of accuracy to prevent a surge in false positives - a common headwind which usually impedes deployments of AI-driven products.
However, cyberspace’s unique characteristics, which have positioned it as a hotbed for fraudsters and malicious actors, aggravate some of the fundamental challenges around the maintenance of data quality, affecting the reliability and performance of AI-based cybersecurity systems.
Whether it’s the emergence of ‘data poisoning’ attacks, or the existence of legacy errors and ‘unknown unknowns’ that lurk within seemingly curated and cleansed training datasets, one thing is clear: validating and preserving data quality in cyberspace is a Sisyphean task.
In this context, one particular and perhaps non-intuitive challenge that security professionals could be facing is the existence of AI bias, as ‘sensitive’ attributes, such as gender, might be misrepresented in datasets used for security purposes, even in the absence of malicious intent.
It is true that any security-related data point, including a representation of a human attribute, could be boiled down to the bits and bytes that compose it; however, the premise that legitimate human-driven activities in cyberspace could be discerned from non-human and malicious digital behavior, implies that human attributes might still matter.
Therefore, as cybersecurity and anti-fraud vendors choose to leverage user-generated content (e.g. e-mails, biometric data, etc.) to spot, for example, malicious or fraudulent activity, AI bias could surface, eroding the efficacy of seemingly impartial security controls.
Trust and governance
The pivotal role of AI models in modern security stacks dictates that quality assurances vis-à-vis the underlying data that organizations and security vendors opt to consume, would remain a prerequisite for sustaining a robust organizational security posture. ‘Zero trust’ is not applicable in this regard.
Security datasets should be carefully curated, minimized, processed, and monitored, kept up to date, and tied to relevant context, as definitions and scopes usually evolve over time, especially in industries such as cybersecurity, which are engulfed in fluid marketing and technological terminologies.
The lack of proper governance that takes into account matters such as data veracity, integrity, provenance, distribution, and privacy, could render security datasets obsolete, misleading, biased, erroneous, and even suspectable to ‘data poisoning’ attacks.
Looking into the future, the cyber security industry should conjure up innovative methodologies and technologies that will enable organizations to establish sufficient trust in the training, validation, and testing datasets that are ingested by AI-bases security models. Otherwise, organization will suffer from a systemic lack of confidence in the performance of their critical AI-powered security controls and processes, which might lead to dire operational and business consequences.
Eyal Balicer is Senior Vice President, Global Cyber Partnerships and Product Innovation at Citi