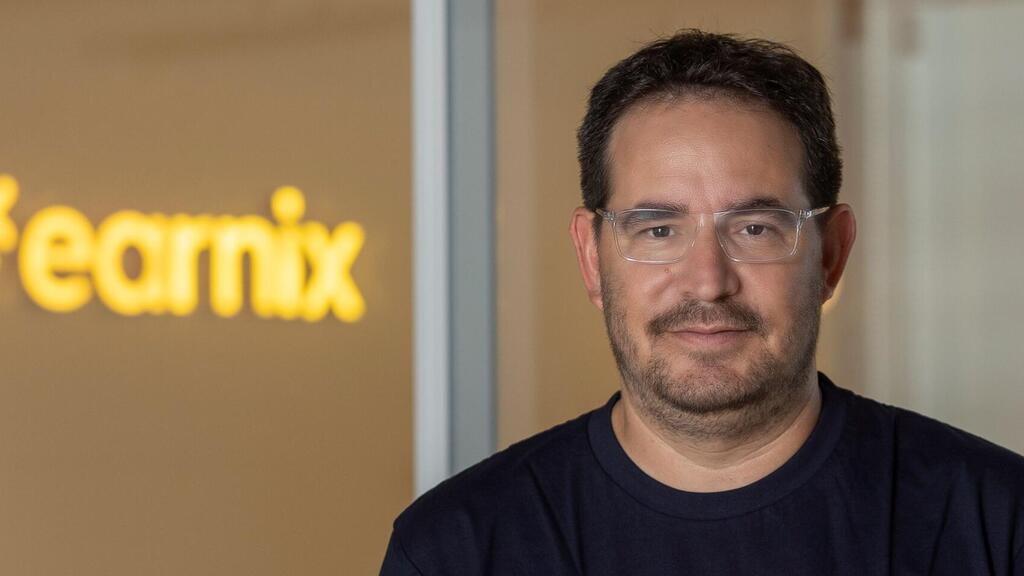
Opinion
Ethics in AI: Moral or commercial interest?
“The ethical challenge in AI models is complex, and their implementation requires companies to make significant financial investments. However, the task is within reach for any company,” writes Earnix CTO Erez Barak
International regulation surrounding the ethical use of artificial intelligence is expanding. Experts are looking to address the fundamental issues of fairness and equal opportunities involved in the use of AI. In March 2024, the United Nations General Assembly unanimously adopted its first global resolution on AI, which aims to ensure that AI benefits all of humanity by promoting its ethical, secure, and inclusive development.
This type of regulation represents a positive moral development, compelling companies and organizations to build and implement ethical, transparent, and fair AI practices, but it also offers numerous commercial benefits. It comes in response to unequivocal demands from consumers worldwide. A survey conducted in the retail sector in January 2024 revealed that 90% of consumers believe that networks should be required to disclose how they use data in AI applications. Additionally, 87% believe that consumers should have access to the data networks have collected about them and be able to review it. Finally, 80% believe that networks must obtain explicit permission from them to use their data in AI applications.
Today, organizations understand that ethical AI is not merely a moral issue but a strategic commercial advantage. By implementing ethical AI practices, companies reduce or eliminate regulatory risks while also building trust with customers who demand transparency and responsibility. While commendable in theory, the task facing commercial companies to achieve this ambitious goal is complex. For example, auto insurance providers collect vast amounts of data, including demographic information about clients, claim histories, vehicle information, property details, and more. AI algorithms, such as machine learning models, analyze this data and identify patterns and correlations, allowing insurance companies to more accurately assess risk and price policies accordingly. The challenge is that the types of data collected about customers are diversifying and increasing all the time, including age, gender, area of residence, driving behavior, and more. While this enhances the ability to assess risks and offer personalized policies, it also increases the risk of biases and discrimination. Variables that seem neutral on the surface may cause various biases and discrimination. How can we ensure that identifying a driver's area of residence does not automatically increase their risk level and raise their policy price?
The Interest in Preventing Bias in AI
To address biases in AI, fair practices must be embedded in the decision-making processes, with multiple layers systematically applied in algorithms across various fields, including banking, insurance, academic institutions, the military, and security organizations evaluating candidates, among others. Some of the parameters worth considering for adoption include:
- Demographic parity, which neutralizes sensitive personal information in the automatic decision-making process,
- Equal opportunity, aiming for similar positive outcomes among different population groups,
- Predictive equality, ensuring a similar rate of negative outcomes among different population groups, and
- Equalized odds, combining all these elements and applying equality in both positive and negative outcomes. Fairness at the individual level should ensure that different people receive similar predictions regardless of irrelevant personal characteristics.
Implementing all these components will enable companies to prioritize and measure fairness in their AI models, including in segmentation and metric selection. It is not just about identifying disparities but taking tangible steps to address them.
Indeed, the ethical challenge in AI models is complex, and their implementation requires companies to make significant financial investments, managerial attention, technological adjustments, and customer training. However, the task is within reach for any company building AI models. Ultimately, anyone who has built an AI model can use their knowledge, tools, and practices to make the necessary changes, just as data scientists do continuously in regular business activities. Compliance with AI ethical regulation is now a top priority for both companies and millions of customers.
Erez Barak is the Chief Technology Officer at Earnix.