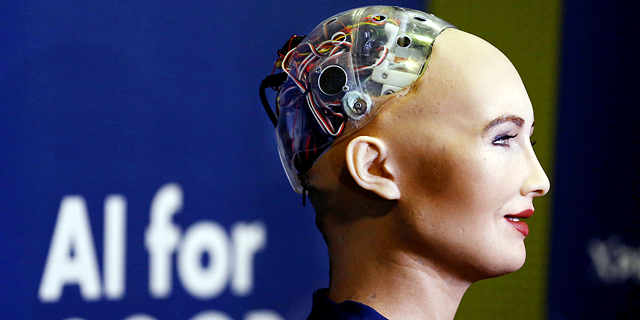
Four Considerations for Making AI Sustainable
For AI to be successfully integrated into the world, we must look beyond the strength of teams and the developments of the technology
My latest project has taken me deep down the rabbit hole of the world of AI, and I want to share with you a summary of all my findings. What follows are four musts for any startup dealing with AI to be tenable.
For founders, if these things are not honed and solved, go back to the drawing board.
For investors, if these things are not honed and solved, reconsider.
1. Defined Use CaseAI is all about prediction — using the information you have to generate information you don’t have — like predicting if a medical image is precancerous, or if a car should slow down if there is a pedestrian.
AI is at its best when the use case is determinate, and based on a defined process. Broad use cases need to be broken down into manageable steps to be successful.
Make sure you can monopolize a particular use case. If you can’t, keep narrowing down your niche.
2. Proprietary Data
Google, Facebook, and Amazon own troves of data. That is why these companies are leading the AI push.
Once the use case is defined, it is imperative to own the data for the niche or the vertical. There are plenty of open-source data sets available for testing, but this isn’t good enough. When everybody has access to the same information, there is no unique value proposition.
One option is to find the partners who have access to relevant data very early and lock them in. Zebra Medical, for example, built partnerships before developing a product.
You can also get creative. X.ai is using AI to create virtual personal assistants. The company's team worked as personal assistants themselves to build the proprietary data required to train the algorithm.
3. Solving the Last Mile
Data is only as valuable as how it can be used to deduce actionable information. Once the use-case is defined and the data is secured, it must be packaged in a way to give insights that can be converted to actionable recommendations.
“Middleware” is simply not good enough for the world of AI.
4. Securing Buy-In from End-Users
It is one thing to build an AI product for a technology multinational, another to build an AI product for your accountant. While the first has the techniques and tools to integrate AI products into the relevant workflow, the latter may still be sending faxes.
For an AI product to succeed, it must have secured buy-in from the end-users. Understand where the product integrates to the customer, what tools are available and necessary. Work with potential customers to bring them to a position where they understand the potential benefit and are willing to test products.
This article was previously published on Medium.
No Comments Add Comment